Trustworthy AI for Automating Ultra-Large Network Optimisation
We aim to develop trustworthy and explainable tools for resource allocation decisions. Our Approach includes utilizing probabilistic inference for interpretability for applications that involve control functionality (e.g. UAVs). Here reinforcement learning is combined with stochastic model predictive control and formal methods to guarantee decision transparency and convergence. This work also involves studies in symbolic AI and neuro-symbolic AI approaches to creating interpretable explainable RL models and the exploration of symbolic machine learning and ILP-based learning to enhance robustness in dynamic resource allocation in RAN slicing.
Formal Verification of 5G advanced protocols to inform a verifiable 6G
As O-RAN adoption continues to expand, AI/ML-driven applications (xApps) are increasingly being deployed to enhance and simplify network management because of their effectiveness in dealing with the complexity and the rapidly evolving nature of real-world network environments. However, AI-based applications can introduce new critical challenges that traditional models did not face, such as the risk of misconfiguration by introducing logical inconsistencies when trying to balance between various QoS. Exhaustive testing is not always an option, however formal verification can identify these inconsistencies and therefore provide a level of guarantee that such dynamic systems are safe, secure, with optimal performance before deployment.
Privacy-Preserving Technologies in 6G
Modern networks collect sensitive user data, raising privacy concerns. Data aggregation alone is insufficient for safeguarding privacy. Through the development of methods for automated privacy leakage evaluation under various attack scenarios we can estimate privacy leakage through adversarial optimization, assessing the effectiveness of complex defence combinations.
New Adversarial Threats and Post-Quantum Security
This project will apply multiple encryption methods to develop a robust and time-efficient hybrid quantum cryptography framework (HQCF) to provide robust protection for the plaintext message and resist cyberattacks. This work involves wireless continuous-variable QKD for IoT devices, MIMO Quantum Key Distribution (QKD), and Hybrid Quantum Cryptography framework for Secure Wireless Data Transmission.
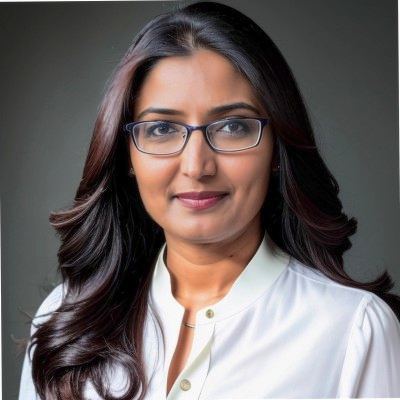
Lead Contact:
Dr. Poonam Yadav
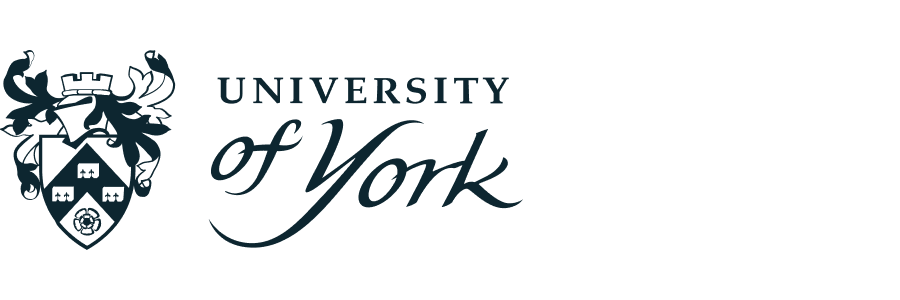
Email: poonam.yadav@york.ac.uk
Address:
Department of Computer Science
University of York
Deramore Lane
York YO10 5GH
United Kingdom